Collaborative automation technology surges into industrial manufacturing
Mike DeGrace, ecosystem success manager—Americas at Universal Robots, will present “Emerging Trends in Collaborative Automation" during A3's Automate Show in Chicago's McCormick Place on May 6 at 8 am. Contact him at [email protected].
Advancements in collaborative robots (cobots), growing digitalization of industrial processes and the emergence of artificial intelligence (AI) make 2024 an exciting time for manufacturing companies exploring their automation options. Some key trends and nascent possibilities are making the collaborative automation space inspiring.
Over the past decade, the industrial sector has enthusiastically embraced cobots, driven by these robots’ ease of use, affordability and versatility.
Cobots were initially designed for human-scale automation but are now adapted for higher payloads and larger scales, pushing cobots further into the industrial market and opening more applications, including heavy-duty palletizing and machine tending. These robots need different kinds of end-of-arm tools (EOATs) than those designed for low-payload cobots, such as grippers with higher force and payload capabilities.
Bigger cobots and AMRs
The trend toward larger cobots is also changing programming approaches and needs. For example, freedrive—a form of programming that involves moving the robot arm by hand—is more limited when dealing with the form factors of larger cobots. Additionally, understanding momentum with masses greater than 10 kg creates new programming considerations.
Safety also needs to be looked at as the working envelope and payload capabilities of these cobots are much larger. Safety standards are evolving to incorporate more considerations for these larger cobot arms and technologies like line area scanners and sensitive skins are being deployed to ensure safe and efficient deployments.
Another empowering trend is the rise of cobots mounted on autonomous mobile robots (AMRs). AMRs differ from the more traditional automated guided vehicles (AGVs) because they don’t require permanent infrastructure, making AMRs adaptable to various environments. This adaptability is due to the various advanced sensor technologies—from ultrasonic sensors used for active ranging, light detection and ranging (LiDAR) and global positioning system (GPS) for detection and localization and gyroscopes for increased guidance performance—incorporated into AMRs.
Providing the ultimate in mobility for collaborative automation, AMR-mounted cobots are being deployed in factories to enable rapid deployments across different cells and to streamline materials transport. In the agricultural sector, these mobile cobots have been used both indoors and outdoors for applications such as fruit inspection and harvesting.
IIoT and AI
The convergence of the Industrial Internet of Things (IIoT) technology, artificial intelligence and collaborative automation is another exciting trend that’s helping to boost productivity.
IIoT is a blanket term for connecting devices—everything from tiny sensors to large cobots—into networks that work together. Integrating machines into IIoT processes allows for easier managing, upgrading and maintenance. One of the most important benefits of IIoT is analytics, which, when combined with AI, enables data analysis to be performed automatically.
Analytics software is being used to monitor cobot performance according to well-established KPIs such as overall equipment effectiveness (OEE). These systems can tell you how many cycles a cobot cell has performed and the speed at which the cobot is performing its tasks, allowing end users to tweak their cobot cells to boost performance.
AI is being used to help improve a wide variety of collaborative processes from unstructured bin-picking to enabling automated, real-time adjustments within cobot cells.
One of the most exciting developments in the cobot space is using AI to provide cobots with some in-built intelligence and understanding about industrial applications. This isn’t analogous to a Level 5 AI, like that found in full self-driving vehicles; it’s more equivalent to lane-guidance technology.
In a screwdriving application for example, AI could simplify the deployment process by having a built-in understanding of the key mechanics and physics of identifying the correct hole, inserting the screw at the right angle and then fixing it into place. That kind of intelligence eliminates a lot of tedious programming.
Conversations about communication
There are different ways to retrieve and handle the vast amount of data collected by IIoT and AI systems. As a result, questions about the best ways to collect and transmit this data are hot topics among manufacturers.
While wired connections to devices are proven to be reliable with immunity to noise, a big downside is the physical component of equipment needed. Meanwhile, wireless connections offer greater versatility, range and ease of setup, but do have more vulnerabilities to inconsistencies.
Plants are evaluating Bluetooth, Wi-Fi, Zigbee, and 5G for communication between devices. Bluetooth performs well for some applications, but it is less data-transferrable compared to Wi-Fi or Zigbee due to energy restrictions. Bluetooth is also susceptible to interference. Wi-Fi has great potential for machine sensors in factories, due to its fast setup and high bandwidth for data transfer. And Zigbee performs extremely well when used on battery-operated sensors with low data throughput.
Meanwhile, 5G is one of the most promising technologies for manufacturers as it provides some real advantages over 4G systems, including enhanced mobile broadband and channel capacity, lower latency and extremely fast reaction times. Critically, 5G can support the transaction speeds required to enable AI and IIoT applications.
On the edge
Another important trend is seeing manufacturers offload processing from local devices to servers outside the plant. While this reduces the amount of processing that local devices perform, it can present cybersecurity risks and consume a lot of time and bandwidth.
Edge computing’s goal is to keep processing as close to the “edge” as possible, thus making analysis faster, more efficient and more secure, since your data never leaves the factory. The edge AI concept allows AI computation to be done near the user at the edge of the IoT network, instead of in the cloud. This brings real-time intelligence to industrial processes, increases privacy and enhances cybersecurity, while reducing costs and securing persistent improvement of the manufacturing process.
A report from the U.S. Energy Information Administration revealed that around 33% of total U.S. energy consumption can be attributed to the manufacturing sector. With sustainable manufacturing another trend to watch, companies have been using IIoT energy-optimization sensors to monitor the electrical status and usage of devices and machines in a factory, enabling operators to fine-tune the process and automatically optimize energy usage.
Digital twins
In the real world, time is a fixed variable. But with digital-twin software, time becomes immutable, allowing you to train real-world applications faster. Digital twins provide an always on and up-to-date computer-simulated version of real-world IoT-connected physical things, people or processes, including collaborative automation.
They can provide synthetic training data for AI models and use sim-to-real processes to better train real-world applications. IIoT enables connected machines and devices to share data with their digital twins and vice versa.
Cybersecurity and blockchain technology
As companies deploy more IIoT devices, remote monitoring and AI into their production processes, new cybersecurity challenges emerge. A trend that I expect to see grow in the future is manufacturers using blockchain technology as a secure, tamper-proof system for transactions and data management across industries.
The blockchain provides a complete, verified, immutable record of all transactions among a set of users. This technology helps manufacturers streamline business operations, improve supply chain visibility and better manage assets.
For example, smart contracts could be used to execute payments automatically when specific conditions are met. A smart contract could automate payments based on an existing service agreement, so that a maintenance provider is paid automatically from a digital wallet when it performs a maintenance operation on factory equipment.
These advancements in collaborative automation, aligned with IIoT and AI, increase automation flexibility and precision and boost productivity and quality. Ultimately though, they are laying the groundwork for an extraordinary future in which human creativity is enhanced by robotics.
Continue Reading
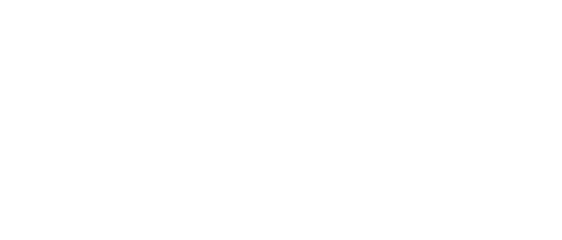
Leaders relevant to this article: