Cognitive is the next stage in robotic development. It is defined as being, relating to, or involved in the conscious intellectual activity. How might it affect the future of machine building? Is this really an application of artificial intelligence (AI)?
I walked around Automate 2024 looking for an artificial-intelligence application that I felt had sustainable qualities. Many of us have followed vision products, and one can say that the AI application in vision, machine learning, is applicable to building machines. I stumbled upon the Neura Robotics booth and talked to one of the research people just out of curiosity and then received an email to talk with CEO David Reger.
He says that we cannot design the same way we always have. The Neura business model is not about just creating a robot, but making robots more interfaceable in a human world. They are developing their own ecosystem, which allows them to be drivers to advance robotics with industry partners such as Kawasaki and Omron.
Is Neura creating a new classification of robots?
Neura answered with cognitive robots that can correct themselves without disturbing production flow. Pick-and-place is a current example because of the market driven by Amazon and other logistics companies and because vision applications using AI are the furthest ahead.
However, learning times used to be an issue. Also, there are the debates about safety and differences in hardware and costs.
What has been done to decrease the learning time for AI applications?
Five years ago, learning times for AI were longer. In response to long learning times, Neura created an incubator that can be implemented locally. The ability to stick objects in “the box” as it learns the patterns allows the controller to learn and distribute information to other robots on the network. Reger also mentions scalability.
This means, if you expand your conveyor system and add a robot, then you just upload the AI that the other robots are using and merge that robot into the system. Instead of six axes, you can have 36, if that matches your application.
The industry trend to create plug-and-play applications is at work here. The software application and architecture are key.
Is the software different?
Software in a Neura system has three levels: sensor, central control or edge and cloud.
From a programmable-logic-controller (PLC) standpoint, think remote input and output. Neura applications allow machine builders to add as many robots as they need. Because one controller has contact with every robot in the system, each robot knows where they are in space, and they can be programmed to do a function together. This allows a type of modularity, though the robot is not changing physical shapes as some other manufacturers are building robots that the user can put together and make organic axis geometry shapes.
Thus, we now have three sets of robot types: industrial, collaborative and cognitive.
Cognitive allows the robot to recover from a mistake and not just slow and back out to a safe zone to rehome. This means the robot adapts to change in box sizes for picks. Changing bin size or allowing the robot to choose its end effector based on what it sees, without doing a tooling change is going to give production areas flexibility. What allows a cognitive response? The software stack and advances in hardware.
Does the collaboration with Kawasaki have anything to do with the hydraulic electric muscle?
Physical advancements are coming from the research-and-development side of robotics interface with Neura software advancements. Robotic companies like Kawasaki and Omron are partnering with Neura because it’s a good combination of classical industrial robot base and research and development that can move more nimbly for changing technology advancements. For instance, the innovation of Kawasaki’s hydro servo muscle using hydrostatic servos allows more humanlike response in a compact form. Neura can use the feedback for movement response. Eventually it will be on the plant floor as a new instrument.
How does your AI application apply to machine building?
In general, servo actuators are being made to put the drive, motor and actuator into one package and in a smaller footprint. Also, there is now adaptive visual servo control. This means that visual feedback and servo position can be read to understand where an actuator arm is in space. The other side to the movement is not only where the arm is in space, but its path plan, obstruction detection and ability to stop.
What are the safety challenges for using a robot with AI?
Are there still safety challenges? Yes. Safety standards are not written to accept an AI type monitor. Builders must be aware of this. Also, there is a fear factor of the unknown. The automation community has not tested AI for as many years as we have tested relays. But, even if a robot makes decisions with AI, it can stop its motion based on a non-AI input. Human comfort is a limiting factor now for AI interface in robotic applications. Think of this like an emergency-stop to say don’t execute a decision.
We are on the edge of bridging these gaps with the onset of smart relays and allowing software-based safety PLCs, but the comfort level is not here yet. Hardware advancements will allow further AI implementation based on a greater capacity to sense the world it’s operating in. The trend will continue to be instrumentation that does more in the field and dumps data back. This means the control architecture is going to continue to change, and software will continue to make it more complex. Hardware will continue to be in flux, as well.
Has force detection impacted design decisions?
Advancements in force sensing has changed the end effector being used in collaborative robots. This is not just for human safety, but for what and how a robot picks something up or grasps a tool. Gripping distances are in millimeters with specific pressure from actuators, using feedback so as not to break products, but allow quality testing. Applications include plastic molded caps and medical packaging that must be secure but come off with specific pressure.
What about the humanoid?
I also asked Reger about a real humanoid “skin.” Ten-plus years ago, I had a conversation with a university professor of neurology about duplicating the neural sequences of a crawfish in software. This is along the lines of the Human Genome Project, except that mapping the human genomes is less complicated than a neural network, as far as volume is concerned.
A crawfish has fewer connections than a human. Keep in mind that the Human Genome Project was launched in 1990. It took 13 years and outlined 3 billion base pairs. Human neural network connections would be in the trillions. Imagine a robot trying to discern touch via a “skin” with hundreds of sensors? The ability to process inputs in milliseconds from instrument to edge is huge.
Possible? Yes. The automation community is still developing material and electrical hardware to make it happen efficiently. Advances in AI and machine learning will be dependent on materials and hardware.
In conclusion, it should be observed that there are two value lines in advanced robotics currently. The research-and-development side that is advancing AI applications in cognitive robotics where the inputs from the environment can be used as inputs to the system to allow the robot to make decisions, and the industrial side that is applying proven functionality to make pick-and-place more efficient, increase the dexterity of end effectors and add cognitive type inputs such as 360° scanning and path planning based on vision and physical position, as well as cell development based on unlimited axis/modularity. The software overhead is large, so costs must be considered. However, costs will decrease and trickle to the plant floor as the technology advances.
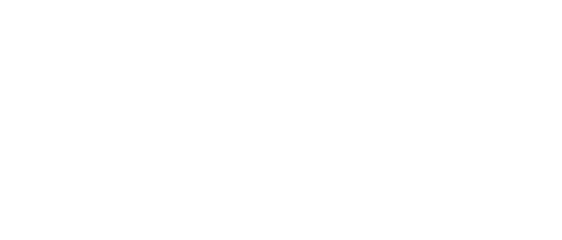
Leaders relevant to this article: