Years ago, out of necessity, we started out with machine vision systems built from PCs, frame grabbers, big cameras with proprietary buses, and bad software. We’ve been able to simplify and move to smart cameras. Some of our sensing applications are only about color and shade, so we see possibly more simplification even though we expect to need to provide more speed and sensitivity going forward. Are there practical differences and limitations between what some companies call smart color cameras and others call smart color sensors? Are we beyond RGB at this point?
– From August ’14 Control Design
Answers:
Lots of Progress
Color smart cameras and smart color sensors both have progressed a lot in the past several years. Starting with smart color sensors, this technology has made big gains. Not only do they have greater color resolution, many manufactures also offer versions of these sensors that can give calibrated RGB color results. Traditional color sensors output only a "match/no match" condition, but some devices can output the analog values for each RGB reading. Now machines and operators see not just which color, but how much color—enabling tighter control. This level of technical expansion also has happened in camera-based color sensors. The ease of getting color images and actual color data from these images has made camera technology a much more approachable solution. Many manufacturers offer simple tools to get the data people are after. Some manufactures also offer even more advanced inspection devices like 3D cameras with tools to capture color data simultaneously with 3D data, and then overlay the two images to make realistic reconstitution of parts even more detailed, so that inspections can lead to more finite detection of color, and high-resolution 3D can lead to detection of previously undetectable flaws in parts. All these things can be done in devices with simple Ethernet connections, so no need for special hardware, which makes these new options for color even more cost efficient.
Jim Anderson,
Product Manager–Vision and 2D Code Readers,
Sick
Go With Color Sensors
In the past, color sensors emitted light using red, green and blue LEDs. The sensors could distinguish colors from the sensors’ reflected light using the RGB components of the reflected light. As technology has progressed, true color sensors have been developed that not only can compare colors, but measure them more accurately than the human eye.
True color sensors typically use white LEDs that provide a consistent white balance. Combine this with the CIELAB [International Commission on Illumination or French Commission Internationale de L’éclairage] color system, which is one of the most versatile color systems, and the result is a color sensor that exceeds the human eye. The CIELAB color system is a three-dimensional representation with the L component for lightness and a and b components for color.
Also Read: Add Vision to Robots — See the Difference
Due to the technology, a true color sensor can check only a small spot of color, but it can check this spot amazingly fast—up to 1.5 kHz with a range of 400 mm. Unlike the color sensor camera, which will focus on the object’s surface pattern and might cause false readings, the true color sensor will ignore patterns, thus providing more accurate color detection. In addition, the true color sensor will have more outputs than the color camera.
Smart color cameras work with RGB but also work with HSV color models. They can be used to check larger areas for the same color or color codes on a part, but have a slower update rate of 50 Hz. Special cameras for faster applications exist but at higher costs. It is important that the light source for the smart color cameras be a white light with a standardized white balance, and this light must be kept constant for all checks to avoid errors.
The sophistication on the front end of the color sensor can be much more advanced and still remain a cost-effective option for industrial use due to the fact that a camera requires a much larger processing system. The more sophisticated the sensors are in the camera, the more robust the processor must be in order to process or map the data into an image.
Jack Moermond, Object Detection Marketing Manager,
Mark Sippel, Project Engineering Manager,
Heiko Mahr, Product Marketing Manager, Vision,
Balluff USA and Balluff GmbH
A major difference is that a smart sensor measures color and shade in a single point on a product, while a smart camera measures color and shade at an array of points. Some vendors use the term smart sensor to mean a smart camera with limited capabilities.A color sensor measures color using three or more photosensors with different spectral responses. A smart color sensor might use comparators on the photosensors’ outputs to make a pass/fail decision based on color and shade. More sophisticated smart sensors can be trained to identify different colors.A smart sensor is OK if you monitor, say, the color of a bottled drink, but is useless if you need to measure color over an area, say, to check the dimensions of a colored bead of glue. For color area or pattern measurements, you need a smart camera. Smart cameras have more capabilities than a smart sensor, such as better color classification or the ability to use "reference patches" to computationally compensate for lighting changes.Most smart sensors and cameras still are based on RGB photosensors. Here are two ways to move "beyond RGB." First, they can have more than three photosensor types, and these can have different spectral responses than RGB. For example, the camera can have CMYK photosensors for use in printing applications. Second, they can computationally convert RGB photosensor responses to other color spaces such as HIS or YUV, or a CIE color space if the lighting is controlled. Our BOA smart camera can convert RGB to a color space that makes product defects more visible, and then use sophisticated algorithms to classify defects in that color space.
Ben Dawson,
Director of Strategic Development,
Teledyne Dalsa
[We received these responses after posting the problem to LinkedIn’s Machine Vision Group.]
Needs Three-Chip Camera
In my experience, pretty much every application that needed color analysis (from an integrator’s opinion, rather than the customer’s) needed a three-chip camera. Typical examples are LED color checks in automotive dashboards. When the LED is lit, a single-chip color camera generally struggles to tell the difference between red, orange and yellow. On the finer scale of colors, you really see the difference. Some colors particularly, such as copper, look yellow or orange on a single-chip camera, but realistic on a three-chip.
We've always used a lot of analog I/O to capture process variables. Electrical noise is becoming a bigger headache for us and our customers as the machines' locations co-exist with a lot more legacy electronics, including old drives and motors, power supplies, as well as just an increased density of devices everywhere. We already use digital I/O via EtherNet/IP (and sometimes other fieldbuses) more frequently for other types of machine signals to save on wiring, gain some device intelligence and increase system flexibility. Are we better off just moving entirely to digital I/O?
SEND US YOUR COMMENTS, SUGGESTIONS OR SOLUTIONS FOR THIS PROBLEM. We'll include it in the December '14 issue, and post it on ControlDesign.com. Send visuals if you'd like—a sketch is fine. Email us at [email protected]. Please include your company, location and title in the response.
HAVE A PROBLEM YOU’D LIKE TO POSE to the readers? Send it along, too.
Jonathan Vickers,
Technical Manager,
Stemmer Imaging
Smart Camera Most of the Time
There’s a huge difference between color as in wavelength (spectrum) and color as perceived by humans. This becomes obvious when we consider illuminations that all seem to be white to us (humans). The classic light bulb really emits a continuous spectrum, while modern replacements (tubes, LEDs) only emit a few wavelengths which make up a mix that seems to be white to us. I have some objects here that change color every day—under the sun it is blue, under the modern bulb it is between blue and green. Obviously, from the physical side, only the spectrum is the correct representation—that would have to be a spectrum for each pixel. On the other hand, if the entire inspection is only directed at human perception, then we would need cameras that have filters in front of the pixels, which equal the wavelengths recognized by the sensors in the human eye. I’m not sure that’s 100% the case, but could be built by using multiple greyscale cameras and filters. I think color still is very tough in the complicated cases. Fortunately in many cases it’s simple and a smart camera can do it. In most cases, a smart color sensor is just a smart camera with a Bayer sensor—and still RGB so to say. But I’m sure there are others, too.
Joerg Beutel, MSc.
Hardware/Software/Image Processing,
Atto Systems
A Little Background
There are two basic uses for "color" in machine vision: measuring color to determine whether an item’s color matches specifications and recognizing an item based on its colors. To avoid unnecessary difficulty and/or disappointing results, it’s necessary to distinguish the two.
Measuring color normally involves single-colored items, careful control of lighting and other environmental factors, and careful calibration. It might benefit from quality three-chip sensors or even multi-spectral devices. Where high-speed inspection is required, cameras, as opposed to spectrophotometers, could be necessary. This discussion is concerned with color-based recognition.
What’s important in color-based recognition, whether classification or verification/anomaly detection, is a reasonable estimate of the probability distribution of colors in the selected color space. With such an estimate for each of the classes of interest, one can determine the most likely class and estimate the likelihood that this determination is correct.
Much of the effort to select a specific set of color coordinates has been justified by an attempt to map the colors of the classes of interest into a coordinate system, where the color distribution for each class can be well represented by a single distinct mean value with a Normal (Gaussian) or rectangular probability distribution. This allows the use of simple statistical comparison tools developed over two centuries ago before the advent of computers. Such a mapping, RGB, HSI, HSL, could be useful in some circumstances, particularly when one wants to select a single component for analysis by conventional monochrome machine vision tools. However, for images of items that appear as more than one color, the assumptions of the simple distribution rarely hold. In such cases, reliable recognition results are unlikely or difficult to achieve.
Single-chip color cameras with Bayer filters significantly increase the complexity of color distribution in the resulting image. Abandoning the assumptions of simple color distributions in favor of arbitrarily complex distributions causes the errors that inevitably result when the simple assumptions don’t hold to disappear. The cost is much more computation, impractical in Gauss’s time, but straightforward today with modern computers and carefully crafted, efficient algorithms.
Robert McConnell,
President,
Way-2C
[We received this response after posting the problem to LinkedIn’s Industrial Automation and Process Controls Group.]
Still About RGB
Most color cameras, smart or otherwise, use a Bayer filter over a monochrome sensor to derive color. That filter consists of one red, one green and two blue pixels, and then the software converts that pattern into an image that we can understand.
A Bayer filter effectively lowers the resolution of the sensor. The only single sensor that doesn’t use this approach is the Foveon sensor, which uses three layers and, to the best of my knowledge, has not made it into machine vision cameras. For higher resolution color, the current approach is to use three very carefully aligned sensors, one with a red filter, one with a green filter and one with a blue filter, and a set of prisms to split the incoming light so that it strikes all three sensors. In the color realm, things are still RGB.
As for the difference between smart cameras and smart color sensors, I have four examples: Cognex InSight 5100C, the new Checker 4G7C, an Xrite sensor, and a Keyence color sensor. The InSight 5100C is a 640x480 resolution color sensor and a full vision tool set in a smart camera, where a smart camera is defined as containing a processor, sensor, network interface and very limited discrete I/O. Checker 4G7C is a 752x480 resolution smart camera with a very limited set of vision tools. The Xrite is basically a camera with a controlled light source, and is able to very accurately verify a color. The Keyence color sensor also uses controlled lighting, and is able to identify up to six taught colors that are fairly dissimilar. Cost-wise the InSight 5100C is around $6,000; I would guess the 4G7C is around $2,000; Xrite, last I checked, was around $4,000; and the Keyence color sensor was around $700. There are other offerings out there with different price points, and my experience has been that the lower-priced offerings impose more limitations.
Randy Zeitvogel,
Vision Specialist,
C & N Manufacturing
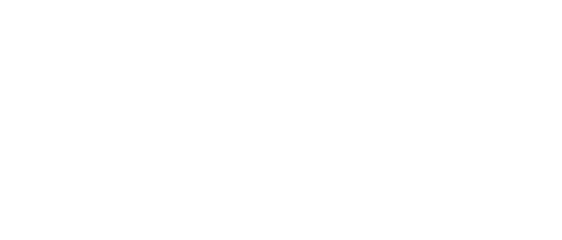
Leaders relevant to this article: